Interview With Dr. Greg Nicola, CMO
- Max G
- Feb 10, 2024
- 12 min read
Updated: Sep 9, 2024
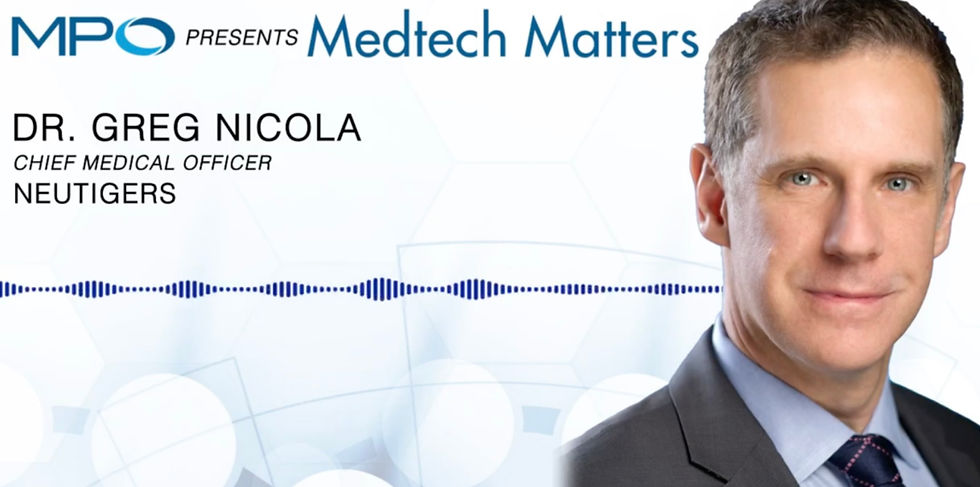
An interesting Interview With Dr. Greg Nicola, the chief medical officer at NeuTigers by Med Tech Editor-in-Chief Sean Fenske
Topic: AI In Healthcare: Interview With Dr. Greg Nicola, CMO
First aired on 5-19-21 Listen on Med Tech website
Sean Fenske
Hi and welcome to another episode of the Med Tech matters podcast where we learn about someone’s impact in the medical device industry. I’m Sean Penn, editor in chief of NPR and host of the podcast. Today we’re speaking with Dr. Greg Nicola, the Chief Medical Officer at NeuTigers. Dr. Nicola, thanks for joining us. How are you doing today?
Dr. Greg Nicola
I’m doing great. Thank you for having me excited to talk about what we’re doing.
Sean Fenske
So let’s do just that. Can you tell me what is NeuTigers?
Dr. Greg Nicola
NeuTigers is an artificial intelligence application company that’s a spin-off of Princeton University. The intellectual property, which are specific, very cutting edge neural networks, is only licensed to NeuTigers. And all the innovations made by the Princeton research lab are licensed to NeuTigers. The uniqueness of the specific neural networks I’m talking about is that they’re so compact, they can work on edge devices. So they can be put on a watch or a telephone, or a sensor inside a foot, like a shoe. So they’re so tiny that they can be placed anywhere, and they don’t need cloud access to make an inference. They’re so tiny that they don’t even need to access the cloud to make any decision tree that they’re programmed to do.
Sean Fenske
What is that? Is that what is meant by when I read the few things we’ve said that NeuTigers is shrinking AI. Is that kind of what that means?
Dr. Greg Nicola
Yes, that’s exactly right. We’ve made AI, not in a cloud-based application, but instead an edge-based application. And you can imagine, well, maybe not a lot of industry needs edge-based AI that you know what cloud is perfectly adequate. But some have security concerns and latency concerns where decisions or inferences have to be made very rapidly, or that the security is so vital that sending additional data of the cloud really doesn’t make sense for that specific type of industry. And, of course, the one we’ve targeted is healthcare.
And let’s get into that; let’s see how, how this technology is being used in the healthcare space.
Dr. Greg Nicola
We beta tested a number of products and in research with a number of others, what we’ve done is we’ve deployed these tiny neural networks into at home monitoring devices and wearable medical devices. And we’re agnostic to the manufacturer of the specific hardware, we can integrate with just about anything, either on the device itself, or just do a simple Bluetooth connection to a cell phone and an application on the cellphone. And by monitoring or putting neural networks on these devices, we can make inferences about the patient’s health very rapidly. And if you don’t mind, I’ll give you an example of one of the research projects that we have are pretty deep in inside France right now.
Sean Fenske,
Yeah, please do because I think it would help clarify exactly what you’re talking about.
Dr. Greg Nicola
So one of the disease states we’re working with is sickle cell anemia. Sickle cell anemia is a genetic disorder where most of our blood cells in in people without the disorder around, and they smoothly move through blood vessels. In sickle cell anemia, occasionally, they have a crisis. And their blood vessels go from rounded shape to sickle and shape. Those sickle cell type blood cells block the arteries, and they can get strokes and heart attacks and very bad chest pain. And it’s quite a painful condition. The issue with sickle cell anemia is the patients usually know they’re about to have a crisis by how they feel. And they can start some intervention at home, which includes just simply drinking a lot of water or you know, anything, inhaling more clearer or more pure oxygen relaxing are some medications, but often, by the time they have any sense of symptoms, it’s too late, and they have to go to the hospital and be treated more rigorously and an inpatient expensive hospital setting. Well, with wearable medical devices and machine learning inferences. We’re finding we can detect crisis earlier than the patient can, meaning that we can implement therapy earlier, and our hope and we haven’t gotten to this phase of the trial. Our hope is that that earlier intervention will lead to less hospitalizations. And that exact paradigm can be used in many other disease states. So we like to call it the most medical terminology is an acute exacerbation of the chronic disease. That’s our market. So representative examples are sickle cell anemia, congestive heart failure, where someone’s heart isn’t beating quite as well and they start getting swelling everywhere. That’s a chronic condition that has acute worsening events. So in essence, we are creating an early warning system where our software which has neural networks paired with these types of wearable devices, has created a whole new layer of information for patients and care providers to look at to start therapy earlier.
Sean Fenske
So it is the AI portion that NeuTigers is providing you said your hardware agnostic, but you’re dependent on the hardware to have the proper technology such as a sensor or whatever it is, the hardware has to be in place to detect whatever it is they want to that’s that wants to be monitored or, you know, kept track of.
Dr. Greg Nicola
That’s correct. And in some cases, for example, we’ve done research in COVID, in sickle cell and mental health disorders and diabetes. Some, in some cases, the sensors are very similar across those diseases, and others, we add other sensors that might not be built into one device. So an example is one of the smartwatches we use has something called skin galvanization, which is how well your skin conducts. And it’s a very good sign of stress. It also has thermal temperature of the skin has something called interbeat interval, which is the intervals between heartbeats. And that we didn’t feel that was going to be quite enough for some of our experiments. And we wanted to learn what else we could add to that. So we added something called the pulse oximeter, which is another little device you can wear on your finger, and it determines how well your bloods picking up oxygen. And we looked at blood pressures. The uniqueness of neural networks is you don’t necessarily need all those data inputs. And all those sensors to make inferences, I’ll reflect on our COVID-19 project, which early in the crisis, we launched experiments in Italy, where people wore devices and then tried to see if we can build algorithms that could detect this symptomology of COVID. And we were pretty successful. We have peer-reviewed literature out and we’re undergoing emergency use authorization to the FDA. It’s not a commercially available product in the United States yet because of the era application. But what we found is that we can build many, maybe 15 to 20 different types of neural networks for one specific disease. And what I mean by that is, let’s say a patient only has access to blood pressure, pulse ox, and temperature. Well, we have a neural network built for that. It has less sensitivity and specificity than something that’s a full-blown, you know, SIX sensor device. But it certainly has accuracy better than just thermal checks for getting in a building. So we can look at neural networks that are tailored to the specific inputs that that patient may have access to.
Sean Fenske
So is this a technology that you’re working with the hardware vendor or the hardware manufacturer on? Or is this something where you’re licensing the new Tygers technology to the hardware vendor? How exactly does that aspect work?
Dr. Greg Nicola
So we have a different market strategy. And there are certainly vendors, for example, we have a kiosk company in the United States where we’re partnering and launching our technology with them. We have one watch manufacturer in Europe where we have a partnership with but other times we’ve done experiments with watches that we have purchased in the consumer market and use them in our research, and then validated them across similar type of watches by adjusting the sample frequency rates of watches may have different frequency rate for similar types of sensors, and adjusting that then revalidating them in smaller experiments. So we have all those tips, different types of models where we can just buy a watch and figure out a neural network that will work with it to actually partnering with a device manufacturer. And we’re not just isolated to smartwatches. We can use any at home monitoring device.
Depending on the disease state. We’re currently looking at trials with EKG monitoring devices and blood pressure monitoring devices, a whole gamut of sensors that are available to us. And as you know, as a tech person, these medical centers are exploding on the market. Some of them are still untested, but some of them are actually FDA approved and have quite a bit of validation behind them.
Sean Fenske
Look at things like the Apple, you know, the Apple Watch and things like that where the senses are embedded in and they’re getting, they’re still getting approvals for different uses. So I see this as a that’s obviously limited to the Apple Watch. But yours has, what I imagine is a similar technology, but used in any form factor.
Dr. Greg Nicola
Correct. That’s exactly right. And you can imagine you can’t fit every single at home monitoring device on a watch, because the skin contact maybe not be conducive to specific physiologic inputs that need to take, you may have to put devices elsewhere on the body. An example is that we’ve worked with a company that makes implants into souls that looks at different pressure on the foot. And we’re looking at analytics to see can we predict potential overuse injuries or exhaustion or even looking at Parkinson’s disease. So there’s a number of use cases that clearly wouldn’t be a watch use case, they would be some other sensor. And we can combine all those sensors if we need to.
Sean Fenske
And in this technology considered, you know, more of monitoring technology, is it considered a diagnostic? Or is there a little bit of both in there?
Dr. Greg Nicola
Yeah, there’s definitely a little bit of both in there. And I will tell you how the company got started is the founder, who is a research scientist and professor at Princeton University, actually did experiments with a smartwatch and his neural networks, which he’s the inventor of, to look if he can actually diagnose disease, not monitor disease, but diagnose. And he again, has peer-reviewed literature on being able to differentiate patients who are healthy versus patients who have type one diabetes versus patients who have type two diabetes. He was actually able to differentiate all three cohorts with a neural network. He actually then launched into mental health disorders. And believe it or not, he was able to do this and mental health disorders. And this was several years back. And when the COVID crisis hit, NeuTigers was very interested in to seeing if we can actually make inferences on the likelihood of COVID. And, and we have been successful, our data is showing in the 90%, greater sensitivity and specificity with fairly low false positive false-negative rates. And that’s validated against not only nasal PCR, which is the gold standard but also with a world-renowned infectious disease expert, who, you know, there are some cases of COVID that are true cases.
But the nasal PCR doesn’t detect them. Well, we double validate them, not only with nasal PCR, but with infectious disease experts validating that they had the classic pattern of COVID. So and we found ourselves to be extremely sensitive and specific for that disorder as a diagnostic capability, although we would use it more as a screening apparatus, as opposed to a definitive diagnostic apparatus, mainly because that’s probably where the market will allow it.
Sean Fenske
Right. And you mentioned, you mentioned diabetes, is this something that could be, you know, ultimately used as a, you know, glucose monitoring type of application? Does it allow for sensing at that level, or I guess it would depend on the hardware, if the hardware could sense the glucose, then it would be able to be used.
Dr. Greg Nicola
So that’s a pretty big device sector, we have not gone into that. Yet. Our main focus has been the exacerbation of disease. So we could detect somebody who we can’t use that strong of language. We haven’t done it yet. But I can imagine, we can build a paradigm that detects somebody’s going into something called diabetic ketoacidosis, which is a very severe diabetic crisis. We could warn somebody that they’re going to go into that with the proper scientific method and developing the model, it would take some time to develop that. But I’m confident now that we’ve been in mental health, infectious disease, and physiologic diseases that probably a lot of diseases could be. There could be our overriding systems for all of them. But as far as monitoring exactly, because of course, there’s already that’s probably going to be I’m not sure you necessarily need machine learning for that specific case, because you’re probably only looking at one data point, which is blood glucose.
Now, if you were going to try to put a bunch of physiologic signals together as surrogates for the blood glucose, maybe I just don’t think it would be as accurate as doing something that targets just one data point, which is blood glucose, and I don’t think machine learning would be necessary for that.
Sean Fenske
And then and, you know, one area that we haven’t even discussed and I would imagine there’s a huge number of applications or opportunities and that would be for sports and training and you know, physical Kirkness like that whole area. I mean, are you you know you mentioned a working with a soul but that was for You know, Parkinson’s and, you know, for the disease are there are definitely opportunities in the sports, you know, area.
Dr. Greg Nicola
There are huge opportunities. We have definitely knocked on those doors but decided as a startup that we need to focus on a specific market sector. I absolutely see that as a huge market sector at some point. Right now we’re trying to tackle diseases that are affecting people, but certainly monitoring and injury monitoring recovery from injury. Those are all plausible scenarios for neural networks and wearable sensors, not necessarily watch, but any wearable sensor that an athlete can put on their body, we certainly would be interested in doing that. But we don’t want to spread our sin off our main mission. Right now. I will say that where we see our target market is in telehealth and the reason is because clinicians now seeing patients over telehealth might not have that tactile sense of how the patient’s doing because they’re not seeing them in person in their office. And we are theorizing that that physician over over a video conference may be more likely to admit a patient because they’re not as confident of how the patient statuses. But having at home monitoring devices mixed with neural networks, helping that clinician with inferences is ripe to make telemedicine maybe even the de facto way to provide care because of the ability, the convenience to the patient, but also the unbelievable amount of data you could get from those that pairing of at home devices and platforms like this. So that’s our heavy push to partner with telehealth companies and deploy this technology to make us all much cheaper and higher value providers to patients.
Sean Fenske
Yeah, I have to say, as someone who, you know, went through a pandemic, physical, you know, had my annual physical through telehealth, you know, it was almost like just having an opportunity to chat with the doctor about a few things. You know, there was no blood pressure reading, there was no wheat and, and height, there was no, you know, checking of different, you know, parameters or biometrics that that aspect was missing. And I really felt like the physical was, was, was lacking because of that, you know, you know, there wasn’t the lab work done, there wasn’t, you know, a lot of the hands on, wasn’t there. And, you know, it was great to be able to see my doctor, but it seemed like such a large piece was missing that this type of monitoring, certainly, combined with telehealth, would be a huge upgrade.
Dr. Greg Nicola
Yeah, we agree you take a subjective encounter and start making it more objective. And that is absolutely where we think our market strategy is. Right.
Sean Fenske
All right. Well, I know you’ve said you want to you know, you want to remain focused on your, you know, your outlined areas of, you know, the specific areas you already mentioned, but can you give us an idea of what might be on the radar after that initial rollout?
Dr. Greg Nicola
Yeah, so, you know, the one thing we are cognizant of is, making doc diagnostic devices are in essence is screening for disease, it may not be the most efficient use of healthcare dollars. So most of our focus will be at monitoring already diagnose disease, even though we are fairly confident we can make a number of inferences for screening for disease. But after that, we are looking at use cases for looking at early classification of Alzheimer’s disease we’re looking at, certainly we’re looking at use cases in the sports sector, we have had several companies that are not going to mention clients, but several big name clients in the United States who are interested in partnering with us to monitor athletes that has been talked about in our ranks. And it’s certainly of sector we may move into. But that would be after we launched these products that we have in the research pipeline right now.
Sean Fenske
Right? Well, I mean, that’s, it’s, it’s great, certainly very interesting technology, and I’m glad you were able to take some time to share the insights with us. Unfortunately, though, that is all the time we have for this episode of Med Tech matters. I’d like to thank my guest, Dr. Greg Nicola for of NeuTigers for sharing his insights and technology with us. And as always, I thank you the listener for tuning in. So until next time, this has been Sean Fenske, editor in chief of MPO, saying thanks for listening.
Interview With Dr. Greg Nicola
Commentaires